情報・人工知能研究
Network Science and Computational Social Science
Research summary
We produce an enormous amount of data. This data can be used to help us understand the society in different ways from how social scientists traditionally have been doing. Our research takes data sets about human interaction and mobility as a starting point. In collaboration with social scientists, we develop statistical and computational methods to analyze such data sets. We investigate patterns of disease spreading, crime, the dynamics of voluntary organizations and the labor market. We also study the mechanisms behind human cooperation, using lab experiments. Many times, our data driven projects lead to more technical questions. To corroborate our findings, we use simulations. The simulations can lead us further into mathematical modeling and the development of efficient algorithms.
To understand how large systems are organized and function, we often use network theory. This means that we simplify data to a set of people and link them together if the data contains interaction between them. Network structure (deviations from just random connections) can tell us something about how a system develops. If one, for example, notices an overabundance of triangles in a social network, one can conclude that there are some social forces that promotes triangle formation. Dynamic systems on networks are also affected by network structure. If there are relatively many triangles in the network, things (like rumors, opinions, disease) spread slower. Network-based methods are thus a method both to make sense of large data, and to connect it to social-scientific theory.
Some concrete questions we are working on at the moment includes: How can we include information about time (of e.g. when people interact, not only whom they interact with) in network modeling? How can network structure promote cooperation? What can travel routes tell us about the functional organization of cities? How do criminals form teams? Can we predict the displacement of people in disasters? How does the awareness of a disease outbreak spread in a population, and how does it affect the spreading of the disease? How can the we optimize networks of mobile devices by knowing the movement patterns of people?
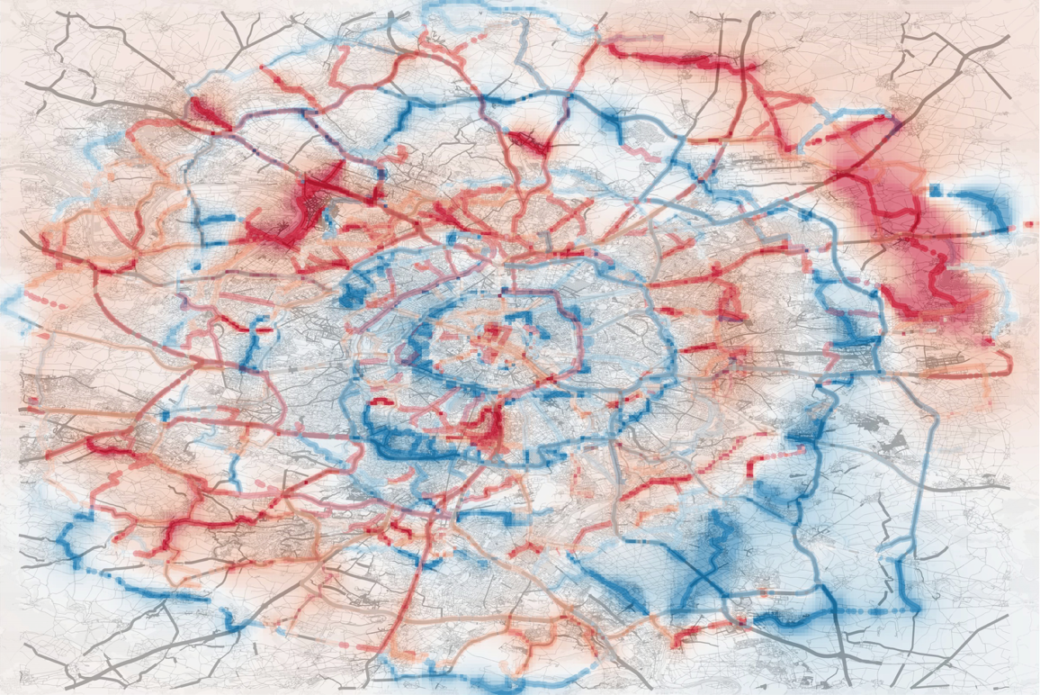
From: M Lee, H Barbosa, H Youn, P Holme, G Ghoshal, Morphology of travel routes and the organization of cities, Nature Communications 8, 2229 (2017).